We know that building trust in technology is a big deal. It’s no longer enough for AI to just work – we need to understand how it works, what it is doing and whether it’s performing as expected.
That’s where model cards come in. If you remember from our previous post, we compared these to the nutrition labels on your favorite foods and snacks. Just as food labels provide crucial information about ingredients and nutritional value, model cards offer essential insights into an AI model’s purpose, performance and potential pitfalls.
So how do you make these “AI nutrition labels” as informative and useful as possible? Let’s dive into the key elements of creating effective model cards and why they are important for fostering trust in AI.
1. Be clear and avoid jargon
When creating a model card, think of it like explaining something to a friend. Be clear and direct. Use simple, everyday language instead of technical buzzwords. Not everyone is a data scientist, so avoid overwhelming people with jargon. For example, if your model has limitations, spell those out in plain terms.
A good rule of thumb is that if a nutrition label can break down confusing terms like “monosodium glutamate” (MSG) into something the average shopper can understand, then your model card can do the same for AI concepts. Clearly defining things like the model’s accuracy, fairness, or any risks helps users understand what they’re working with.
2. Tailor your message to different audiences
Just as nutrition labels are useful for everyone from health-conscious shoppers to busy parents, model cards should be helpful to a wide range of people. Whether developers build the models, business leaders make decisions or policymakers ensure the technology is being used ethically, your model card needs to address diverse audiences. Also, considering cultural contexts can help to avoid misunderstandings and ensure that the model is used appropriately in different cultural settings.
One way to do this is to break down the information into sections that cater to different levels of expertise. You don’t want to throw complex metrics at someone who’s just trying to determine whether the AI model is a good fit.
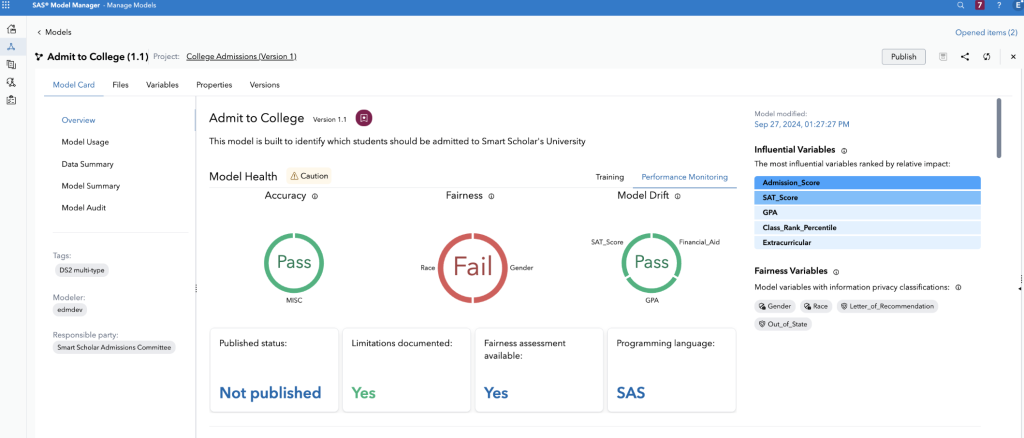
3. Don’t skip the ethics
Ethics in AI isn’t just a “nice to have – it’s absolutely crucial. And your model card is the perfect place to highlight how you’re ensuring your AI models are responsible. As food labels warn us about allergens, sugar content or calorie counts, model cards should give users a heads-up about the ethical concerns tied to your AI.
Include potential biases, limitations, and responsible use guidelines in your model card. Are there risks of unfair outcomes in certain scenarios? Is the model trained on data that might introduce bias? Address these upfront. This kind of transparency can prevent harmful or unintended consequences, ensuring your AI is used the way it was meant to be: for the benefit of everyone.
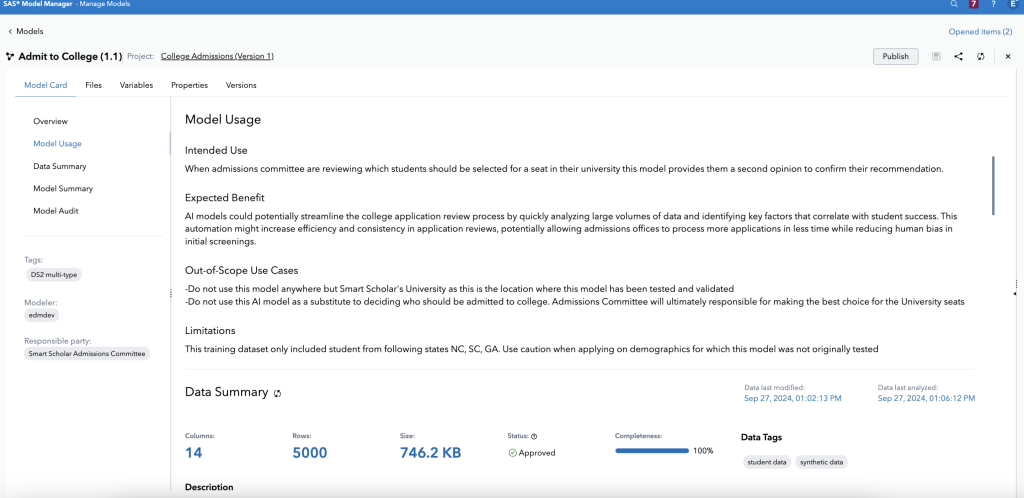
4. Collaborate and seek feedback
Building a comprehensive model card isn’t a solo act. Effective model card documentation benefits greatly from diverse perspectives. Including input from a variety of team members – developers, testers and users – helps ensure the model card covers all the bases and provides a well-rounded understanding of the model.
It’s also key to get feedback from different stakeholders. Is your model card easy to understand for people with varying levels of technical knowledge? Does it address the concerns of those who will be using or impacted by the AI? Seeking feedback ensures you’re meeting the needs of everyone involved.
5. Describe your data
Data transparency is like the ingredients list on a food package – it tells you exactly what’s inside. In your model card, break down the data sources, collection methods, preprocessing steps and any relevant privacy considerations. It’s important to describe the demographic composition of the dataset, including details on age, gender, ethnicity, and other pertinent factors that could influence model performance or introduce bias.
For instance, if you’re using data from a specific time frame or location, mention that. Just as you might want to know if your food is organic or sourced locally, users want to know the origin of the data used to train an AI model. Any known limitations or gaps in the dataset should also be called out, helping others make informed decisions about its reliability.
By applying these principles, anyone looking to use AI model cards can ensure they are clear, comprehensive and helpful for a wide range of people. Remember, just like food labels help us make smarter dietary choices, model cards empower users to make ethical, informed decisions about AI.
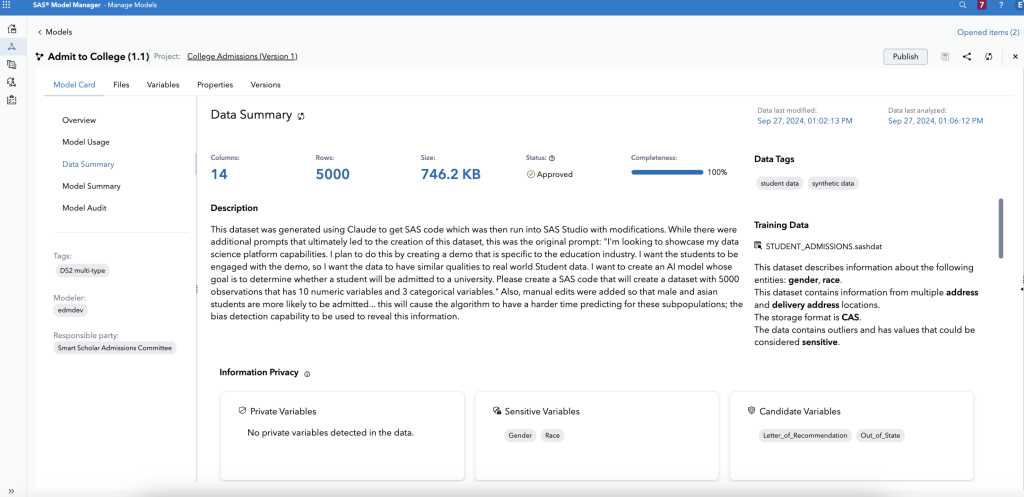
Join us for an Ask the Expert webinar on fostering AI trust using model cards on Oct. 17