Artificial intelligence (AI) is making significant strides in natural language processing (NLP), focusing on enhancing models that can accurately interpret and generate human language. Researchers are working to develop models that grasp complex linguistic structures and generate coherent, contextually relevant responses over extended dialogues. Advancements in this area are vital for applications such as automated customer service, content creation, and machine translation, where language precision and sustained coherence are critical. As the demand for AI capabilities in these applications grows, improving models’ ability to handle nuanced language and maintain context is increasingly essential.
A major issue facing NLP is sustaining coherence over long texts. Language models tend to lose track of long-term dependencies within text, which results in inconsistencies and a lack of context in responses. This limitation is particularly problematic in applications that require extended, interactive dialogue, as responses may need to align with prior context. Resolving this issue is crucial to advancing AI applications that rely on natural language understanding and generation for effective and reliable performance.
Current language models, predominantly based on transformer architectures such as GPT and BERT, have achieved substantial progress but are often limited by high computational demands and restricted ability to maintain context over extended text. These transformers process text in a way that requires significant memory and processing power, making their application impractical in settings with limited computational resources. Further, transformer models sometimes need help with long-text coherence, limiting their effectiveness in complex language tasks. Researchers are, therefore, exploring ways to balance performance with computational efficiency.
Researchers from Amazon and Michigan State University introduced a new model to address these challenges by refining the transformer architecture. This model aims to reduce computational load while preserving coherence over long text segments, employing a novel segmentation approach to maintain the accuracy of contextually relevant responses. By introducing error-aware reasoning through segmenting text into smaller units, the model can process extensive passages without compromising coherence, which is a considerable advancement in the NLP field. This segmentation also allows for scalable modular adjustments, making the model versatile for language tasks, including question-answering and conversational AI.
This model incorporates an error-aware demonstration mechanism, allowing it to adjust predictions based on detected inaccuracies in intermediate reasoning steps. Rather than processing text in one large unit, this model breaks down inputs into smaller segments that maintain contextual links, enabling coherent processing over lengthy passages. The modular design further allows researchers to adjust specific model parameters to match the needs of different applications without necessitating a complete system redesign. This scalability positions the model as a flexible and efficient solution for various NLP applications.
In experiments, this model demonstrated marked improvements across various benchmarks. For instance, in the “Tracking Shuffled Objects” dataset, the model’s accuracy rose from 56.53% to 61.20%, while in the “Penguins in a Table” dataset, performance improved from 81.34% to 82.19%. These results underscore the model’s improved ability to manage complex reasoning tasks. The model also showed significant performance gains on specific benchmarks; accuracy improved by over 2% in some cases, proving that it can consistently outperform standard transformers by accurately managing intermediate reasoning steps.
The study further highlights how the model reduces computational costs while maintaining coherence. For example, accuracy improved by approximately 2% in specific scenarios when applying error-aware reasoning to multi-step tasks. The research found that incorporating correct and incorrect reasoning paths boosted the model’s ability to detect and correct reasoning errors, which is particularly beneficial in complex dialogues or extended reasoning scenarios. These findings suggest that the model’s robust architecture could make it an ideal choice for applications requiring sustained and accurate language comprehension over prolonged interactions.
Overall, this research by Amazon and Michigan State University presents a noteworthy advancement in NLP by addressing critical challenges in maintaining coherence and reducing computational strain. The proposed model balances accuracy with efficiency, promising substantial benefits for various language applications. Its modular and adaptable structure positions it as a versatile tool for real-world AI tasks that demand accurate, contextually aware language processing across diverse fields.
Check out the Paper. All credit for this research goes to the researchers of this project. Also, don’t forget to follow us on Twitter and join our Telegram Channel and LinkedIn Group. If you like our work, you will love our newsletter.. Don’t Forget to join our 55k+ ML SubReddit.
[Upcoming Live Webinar- Oct 29, 2024] The Best Platform for Serving Fine-Tuned Models: Predibase Inference Engine (Promoted)
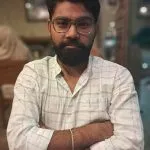
Nikhil is an intern consultant at Marktechpost. He is pursuing an integrated dual degree in Materials at the Indian Institute of Technology, Kharagpur. Nikhil is an AI/ML enthusiast who is always researching applications in fields like biomaterials and biomedical science. With a strong background in Material Science, he is exploring new advancements and creating opportunities to contribute.